A Data-centric Approach to Security and Risk in the Hyperconnected AI Era
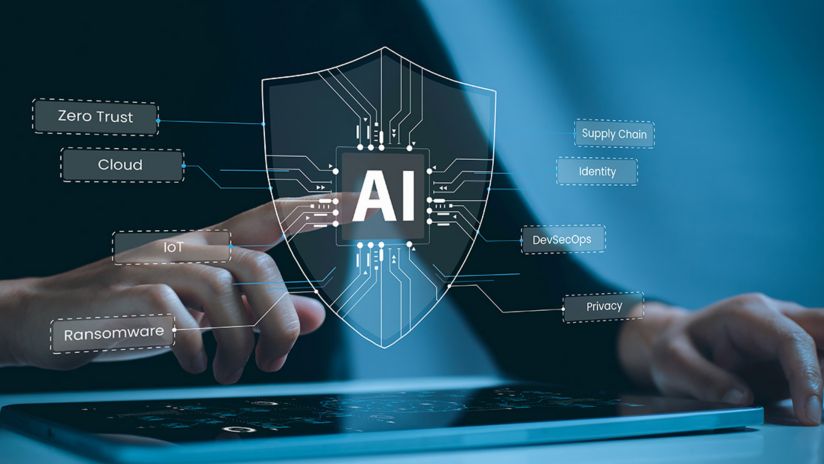
The rapid pace of technological change demands that enterprise and security leaders take a moment to pause and reassess. By studying the trajectory of data sources and data flows, leaders are better positioned to recalibrate their strategies and objectives. This exercise is even more critical for organisations seeking to enhance data security and limit risks to data in this renaissance age of artificial intelligence (AI).
AI has the potential to overhaul every aspect of an organisation, serving as its operating system and transforming the way organisations create value. It will reinvent business models, job roles, and workflows.
Today, many enterprises find themselves largely unprepared for this change. This observation is supported by research from a report titled Generative AI: Differentiating Disruptors from the Disrupted, conducted by MIT Technology Review Insights in partnership with Telstra International.
The report findings reveal that regulatory, compliance, and data privacy issues are regarded as the top non-IT barrier to the rapid adoption of generative AI (GenAI). Additionally, it indicates that 54% of GenAI early adopters acknowledge that their companies’ cyber security measures are, at best, only modestly capable of supporting rapid GenAI deployment.
What’s the way forward? How can enterprises secure data in the hyperconnected AI era?
Short answer: Many companies must evolve their approach to cyber security, data governance, compliance, risk, and privacy.
A Framework for Managing AI Risks
For most enterprises, data flows that drive business value originate from ever increasingly complex and hyperconnected environments. Consequently, enterprise security, compliance, and risk leaders recognise that AI not only introduces new challenges, but also exacerbates existing ones.
This presents a challenge for businesses: They need to swiftly address risks to minimise the loss of business opportunities. According to Gartner, by 2027, 60% of organisations will fail to realise the anticipated value of their AI use cases due to incohesive ethical governance frameworks.
Organisations require a holistic data security and protection model to maintain a flexible, yet robust security posture. Multiple stakeholders managing risk must also act in unison to achieve broad coverage across hyperconnected business environments and digital ecosystems.
Telstra International has developed a comprehensive cyber security framework conducive to the hyperconnected AI era. It takes a holistic approach to data security, compliance and governance, infrastructure, digital ecosystems, and the increasingly rapid adoption of AI technologies.
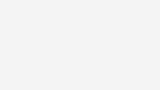
This framework is built around four core tenents:
Zero Trust:
At the heart of this model is a zero trust approach, an essential starting point to protect enterprise assets that are inherently ephemeral and fluid. These include data in motion and dynamic workflows, as opposed to a siloed focus on static workloads.
It is crucial to apply key zero trust principles – including identity verification, least privilege access, microsegmentation, and continuous monitoring and validation – to guard against insider threats and external attacks. An effective zero trust model must encompass users, devices, applications, and services across multiple layers of AI and data stacks – both within and outside corporate networks.
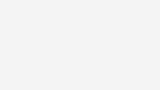
Threat and Risk Management:
In the hyperconnected AI era, enterprises must also ensure they mitigate threats and other risks. The dynamic nature of data in motion, a hallmark of this era, renders traditional perimeter-based security controls obsolete.
Furthermore, Large Language Model Operations (LLMOps) and Machine Learning Operations (MLOps) typically leverage extensive, multifaceted data streams, and workflows that cut across hybrid and multi-cloud environments. This significantly broadens an enterprise’s attack surface, making AI systems more vulnerable to increasingly sophisticated attacks.
Maintaining a robust security posture across an organisation’s digital infrastructure calls for a comprehensive and proactive protection strategy. This strategy is underpinned by a suite of technologies, each of which address various aspects of the data stack:
- Cloud security solutions
- Secure SD-WAN solutions
- Application security solutions
- Identity and access management solutions
- Secure remote access solutions
- Network security solutions
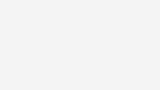
Data Governance and Data Protection (DGDP):
Built on a robust foundation that safeguards the digital infrastructure powering data and AI initiatives, the critical layer of DGDP focuses on mitigating risks. Implementing real-time governance and protection is an important component of digital trust for an always-on business. Key components of DGDP include:
- Data confidentiality
- Data integrity
- Data use principles
- Data access and sharing
- Data governance and compliance
- Data protection
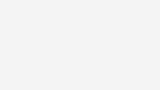
Automation, Orchestration and Expertise:
The security challenges posed by AI-enabled data deployments do not exist in a vacuum. SecOps teams are commonly subjected to a challenging threat vector environment. They face a deluge of uncategorised, disparate and even duplicated alerts from numerous security solutions – often leading to high levels of alert fatigue. These obstacles may impact team morale and also hinder the ability of security practitioners to proactivily hunt, detect, investigate, respond, and remediate vulnerabilities and threats.
To navigate these challenges and enhance the security of AI-enabled systems, security teams must embrace automation, orchestration, and expertise. By leveraging the right solutions supported with expert advice, Security Operations (SecOps) teams can better sift through a fog of alerts to pinpoint critical incidents, and efficiently aggregate and correlate telemetry data.
Combined, these four tenets – Zero Trust; Threat and Risk Management; Data Governance and Data Protection; and Automation, Orchestration, and Expertise – form a comprehensive data security and protection strategy. This framework delivers continuous protection, improvement, and response capabilities that are central to harnessing GenAI and corresponding data safely. In turn, organisations can maximise the benefits of these technologies and thrive in the hyperconnected AI era.
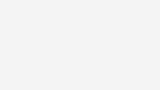
Optimising AI Security With Security Service Edge
Security Service Edge (SSE) provides a strategic model that aligns with many of the objectives of the four tenets. It also helps overcome the complexities associated with securing the rise of AI-enabled multimodal data streams and distributed multi-cloud infrastructure.
Implementing a security and risk strategy built on SSE offers significant advantages for enterprises adopting GenAI and AI, including:
Simpler Architecture:
SSE consolidates multiple security functions, including Secure Web Gateway (SWG), Cloud Access Security Broker (CASB), Zero Trust Network Access (ZTNA), Firewall as a Service (FWaaS), and endpoint DLP, into a single platform. This streamlines security architecture, enhancing the efficiency of managing and enforcing consistent security policies across complex AI infrastructure.
Better Scalability and Flexibility:
Based on a cloud-delivered model, SSE solutions inherently provide the scalability and flexibility required by dynamic data environments. This adaptability is crucial for enterprises that need to scale security measures to align with the rapid adoption of GenAI and AI systems.
Reduced Complexity:
By consolidating network and security tools into a single solution, SSE reduces the complexity of standing up and managing security infrastructure. This reduces the burden on IT and security teams, who already struggle with managing numerous point solutions aimed at protecting data infrastructure—a challenge that only intensifies with the added complexities of AI technologies and infrastructure.
Enhanced Data Protection and Privacy:
SSE helps organisations protect sensitive data by integrating data loss prevention (DLP) capabilities. This safeguards against data privacy breaches and the loss of intellectual property, both top-of-mind challenges of GenAI applications. Additionally, SSE supports compliance with data localisation mandates, as well as sovereign and industry-specific data laws and privacy regulations.
Improved Threat Detection and Response:
SSE platforms utilise advanced threat intelligence and real-time analytics to identify and mitigate threats targeting data and AI infrastructure more effectively from the increasing incidence of ransomware and other sophisticated attacks.
Greater User Experience:
SSE ensures that security measures can be implemented without compromising user experience. By applying network optimisation strategies, SSE can lower latency and boost the performance of AI applications and underlying end-user applications. This leads to a smoother experience for distributed workforces.
Protection Against Generative AI-Specific Risks:
Zero Trust Network Access (ZTNA) is a core component of SSE. ZTNA ensures that all access requests are authenticated and authorised before connections to network resources are allowed. This stringent access control helps mitigate risks such as data breaches, data poisoning, and model tampering.
Staying Secure Amidst an AI Revolution
The performance of AI models follows an exponential growth curve, driven by AI scaling laws. This rapid evolution calls on security leaders to quickly establish a robust data security and protection foundation to keep pace with technological advancements. By adopting a foundational strategy, anchored in the four core tenets and enhanced by Security Service Edge (SSE), organisations are better equipped to secure these transformative technologies today and tomorrow.
Learn more about the state of cybersecurity, compliance, and privacy preparedness among enterprises adopting AI and GenAI from the Generative AI: Differentiating Disruptors from the Disrupted report, a global survey and report from MIT Technology Review Insights, produced in partnership with Telstra International.